Regulations for detecting AI bias effectively
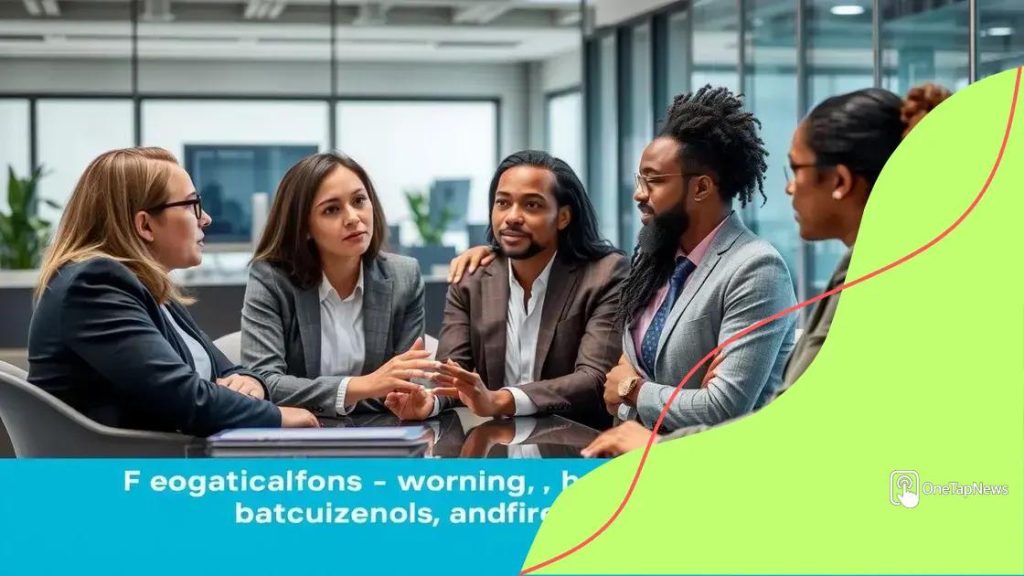
Anúncios
Regulations for detecting AI bias are essential to ensure fairness and accountability in AI systems, guiding organizations to identify and mitigate biases effectively through inclusive practices and continuous oversight.
Regulations for detecting AI bias are becoming increasingly vital as AI technologies evolve. How do we ensure fairness and transparency? Let’s explore these essential frameworks together.
Anúncios
Understanding AI bias regulations
Understanding AI bias regulations is essential for ensuring fairness in technology. These regulations help us identify and address biases that can affect outcomes. They aim to create a responsible framework for AI development and usage.
What Are AI Bias Regulations?
AI bias regulations refer to rules that guide organizations in the ethical use of artificial intelligence. Such regulations encompass a variety of laws and guidelines designed to mitigate bias and enhance transparency in AI systems.
Government bodies and organizations worldwide are increasingly recognizing the need for these regulations. This growing awareness helps to establish trust between technology developers and users.
Anúncios
Why Are They Important?
Understanding the importance of these regulations requires us to look at their implications. AI biases can lead to unfair treatment based on race, gender, or other characteristics. This can result in unjust outcomes in areas like hiring and lending.
- Promote fairness and equality in AI outcomes.
- Establish accountability among AI developers.
- Protect consumer rights and privacy.
- Encourage public trust in AI technologies.
Moreover, regulations can help guide organizations in creating responsible AI systems. By doing this, stakeholders can ensure ethical standards are upheld throughout the AI lifecycle.
Regulatory Frameworks
Various frameworks outline how to manage AI bias regulations. Some key frameworks include the European Union’s AI Act and the IEEE standards. These frameworks provide guidelines to ensure that AI systems are developed and implemented responsibly.
Organizations must adopt these frameworks to align with best practices. Adhering to these guidelines fosters a culture of accountability and ongoing assessment of AI systems.
By understanding AI bias regulations, developers and users alike can contribute towards creating a fairer technological landscape. Their role is crucial in shaping the future of AI, where technology benefits everyone without discrimination.
Key frameworks for detecting AI bias
Key frameworks for detecting AI bias provide essential guidelines to ensure fairness and transparency in AI systems. These frameworks help organizations recognize biases that can disproportionately affect certain groups.
Developing a Robust Framework
Creating an effective framework involves collaboration among stakeholders, including developers, regulators, and ethicists. These partnerships are crucial as they can bring diverse perspectives on bias and fairness.
- Involve diverse teams in the development process.
- Continuously assess and update frameworks.
- Utilize a multi-disciplinary approach.
- Encourage public input and feedback.
Frameworks should be adaptable; they must evolve with advances in technology and understanding of AI bias. This adaptability ensures they remain relevant and effective.
Examples of Recognized Frameworks
Several notable frameworks have emerged in recent years to tackle AI bias. These include the European Union’s Artificial Intelligence Act and the U.S. Algorithmic Accountability Act. Each framework offers unique guidelines and best practices to address bias.
The European Union’s framework focuses on risk management. It categorizes AI systems based on their potential risk to society. Conversely, the U.S. approach emphasizes accountability and transparency among AI developers.
Both frameworks highlight the importance of monitoring bias as technology evolves. This is vital for maintaining public trust in AI technologies.
Measuring Effectiveness
To gauge the success of these frameworks, organizations should implement metrics for assessing AI performance. This includes analyzing outcomes for various demographics to identify any discrepancies.
- Establish clear benchmarks for fairness.
- Monitor user experiences across different populations.
- Regularly report findings to stakeholders.
- Adapt strategies based on feedback.
The key is to ensure that any detected biases are addressed promptly, reinforcing the integrity of AI systems. By leveraging effective frameworks, companies can build systems that are not only functional but also equitable.
Real-world examples of bias detection
Real-world examples of bias detection highlight how organizations address and manage bias in AI systems. These examples provide valuable insights into effective strategies and the importance of vigilance in AI development.
Case Study: Hiring Algorithms
One notable case involved a major tech company that found its hiring algorithm favored male candidates over female candidates. This disparity raised alarms about bias detection. The company implemented measures to correct the algorithm, ensuring gender-neutral language and evaluating candidate scores based on multiple factors.
- Identifying bias through data analysis.
- Revising algorithms for gender neutrality.
- Regular audits of the hiring process.
Such actions showcase how recognizing bias can lead to improved outcomes and fairer hiring practices.
Healthcare Algorithms
Another pertinent example is related to healthcare algorithms used to predict patient outcomes. A specific algorithm was found to under-represent certain minority groups. This disparity showed how biases in training data can impact healthcare decisions.
To address this issue, researchers worked on increasing the diversity of training data and regularly monitoring algorithm performance. By doing so, they enhanced the accuracy and fairness of health predictions across different populations.
Facial Recognition Technology
Facial recognition technology has also come under scrutiny for biased outcomes. Studies have revealed that some systems have higher error rates for individuals of certain demographics. In response, companies have publicly committed to improving bias detection and ensuring their technology performs reliably across diverse groups.
- Conducting external audits of technology.
- Implementing community feedback mechanisms.
- Ensuring diverse data training sets.
These real-world examples of bias detection show that while challenges exist, proactive measures can create a more equitable environment. Through these actions, organizations build trust and responsibility in their AI systems, ultimately benefiting society.
Best practices in AI bias mitigation
Best practices in AI bias mitigation are essential for fostering fairness and accountability in technology. Organizations can adopt several strategies to minimize bias in their AI systems and promote equity.
Develop Inclusive Data Sets
A key strategy in AI bias mitigation is to ensure inclusive data sets. This means collecting data from diverse populations to account for various characteristics like gender, race, and socioeconomic status. Diverse data helps the AI learn more accurately, reducing biases in outcomes.
- Focus on representing underrepresented groups in your data.
- Continuously review and update data for relevance.
- Avoid historical data that reflects previous biases.
By using inclusive data, organizations can reduce the risk of perpetuating existing biases in their AI systems.
Regularly Test Algorithms
Another effective practice is to regularly test algorithms for bias during their development and deployment. This involves evaluating how the AI performs across different demographics. Assessments should be ongoing to catch any biases that may emerge as the AI is exposed to new data.
This process includes setting specific metrics for fairness and creating feedback loops to address any detected biases quickly. When teams are vigilant about testing, they can adapt algorithms to ensure fair outcomes.
Engage Diverse Teams
Engaging diverse teams in the development process is also critical. Different perspectives bring awareness to potential biases that developers might overlook. When teams reflect the diversity of the populations served, they foster more inclusive AI solutions.
- Encourage collaboration across disciplines within teams.
- Implement training on bias awareness and reduction.
- Seek input from community stakeholders.
This practice ensures that various viewpoints are considered, enhancing the quality and fairness of AI systems.
Implement Fairness Guidelines
Organizations should establish and adhere to fairness guidelines throughout the AI development process. These guidelines can include ethical standards and compliance metrics that align with societal values.
By creating a structure based on these principles, businesses can promote accountability and transparency in their AI projects. This not only helps in building trust with users but also minimizes the risk of backlash from biased outcomes.
Future trends in AI bias regulation
Future trends in AI bias regulation will significantly shape how artificial intelligence is developed and implemented. As technology evolves, regulations must adapt to keep pace with changes and challenges.
Increased Global Cooperation
One notable trend is the push for greater global cooperation. Countries are beginning to recognize that AI biases do not respect borders. Collaborations among nations can lead to unified standards and practices that ensure fairness across platforms.
- Sharing best practices among nations.
- Coordinating international regulations for AI.
- Facilitating cross-border data flow while ensuring privacy.
This increased cooperation helps Governments harmonize their approaches to mitigate bias and enhance accountability.
Implementation of Advanced Technologies
Another trend is the integration of advanced technologies such as machine learning and natural language processing in regulation. These technologies can help monitor AI systems in real time, allowing for quicker detection and correction of biases.
Automated tools will enable policymakers to analyze vast amounts of data, uncover trends, and make informed decisions more effectively. With these advancements, regulations can become more dynamic, adjusting swiftly to new findings.
Emphasis on Ethical AI
There’s also a growing emphasis on ethics in the design and use of AI systems. As awareness of bias increases, organizations will be pressured to adopt ethical guidelines. Adhering to these guidelines can help companies build trust with users and minimize bias in their applications.
- Developing ethical frameworks for AI deployment.
- Encouraging transparency in AI algorithms.
- Involving diverse voices in the AI creation process.
This trend towards ethical consideration will lead to a more responsible approach to AI that prioritizes fairness and diversity.
Public Engagement and Awareness
Future trends will likely involve heightened public engagement and awareness regarding AI bias regulation. As users become more informed, they will demand accountability from companies. This shift can pressure organizations to maintain fair practices and adhere to regulations consistently.
Public discourse will also influence policy-making, prompting governments to listen to citizens’ concerns about AI and its implications. Overall, these emerging trends paint a picture of a more regulated and ethically driven AI landscape.
FAQ – Frequently Asked Questions about AI Bias Regulations
What is AI bias and why is it important?
AI bias refers to systematic errors in AI algorithms that lead to unfair outcomes based on race, gender, and other factors. Addressing it is crucial for ensuring fairness in technology.
How can organizations detect bias in AI systems?
Organizations can detect bias by regularly testing algorithms, analyzing outcomes across different demographics, and utilizing inclusive data sets.
What role do regulations play in managing AI bias?
Regulations provide guidelines and standards that organizations must follow to ensure fairness and transparency in AI systems, helping mitigate bias effectively.
What are some best practices for mitigating AI bias?
Best practices include developing diverse data sets, regularly testing algorithms, involving diverse teams in development, and establishing fairness guidelines.